Understanding Asset Condition: Managing Risk and Enhancing Performance with Advanced Analytics
Discover the importance of understanding asset condition for managing risk and enhancing performance. Learn how advanced analytics like machine learning and degradation analysis can optimise your asset management strategy.
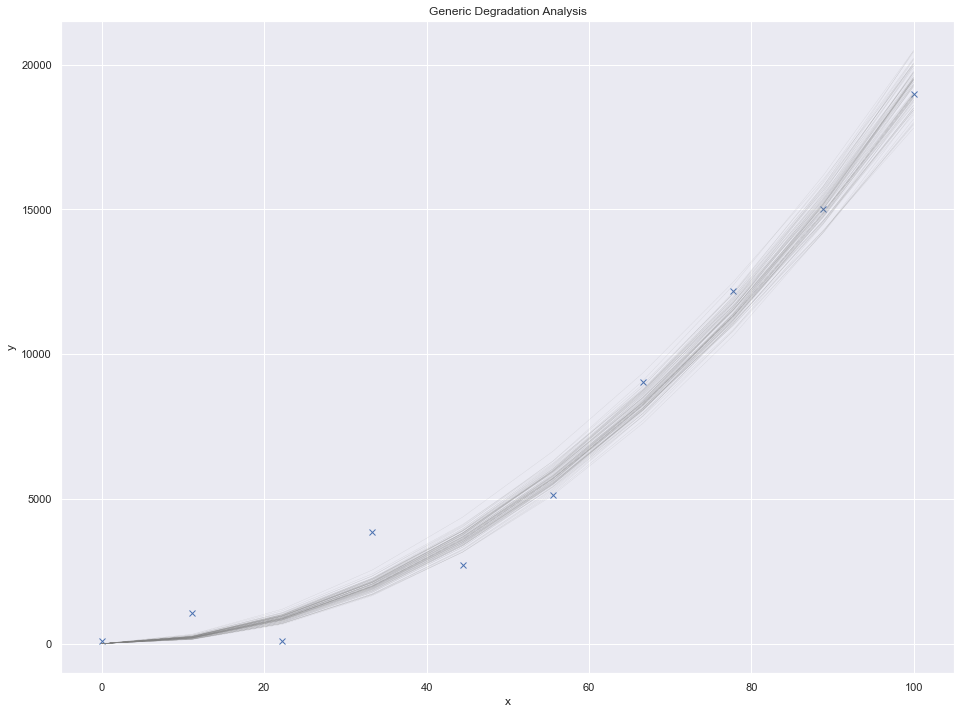
In the ever-evolving landscape of asset management, understanding asset condition is crucial. It is a vital factor in managing risk and optimising performance. By assessing the condition of assets, organisations can make informed decisions, ensuring reliability and longevity. This article delves into the significance of asset condition, explores the role of analytics, such as machine learning and degradation analysis, and provides a practical guide on leveraging these tools.
The Importance of Asset Condition
Asset condition refers to the current state of an asset, determined through inspections, testing, and monitoring. Knowing an asset's condition helps in predicting failures, planning maintenance, and improving safety. It is essential for:
- Risk Management: Identifying potential issues before they escalate reduces the risk of unexpected failures, which can lead to costly downtimes and safety hazards.
- Performance Optimisation: Understanding asset condition allows for timely interventions, ensuring assets operate at peak efficiency and extend their service life.
- Cost Savings: Proactive maintenance based on condition assessments can prevent expensive repairs and replacements, resulting in significant cost savings.
Role of Analytics in Asset Condition Assessment
Modern analytics play a pivotal role in assessing and managing asset condition. Tools such as machine learning and degradation analysis offer advanced capabilities for predictive maintenance and risk management.
Machine Learning
Machine learning algorithms can process vast amounts of data from sensors and historical records to identify patterns and predict future asset behaviour. Key applications include:
- Predictive Maintenance: By analysing data trends, machine learning models can forecast when an asset is likely to fail, allowing for maintenance to be scheduled just in time to prevent downtime.
- Anomaly Detection: Algorithms can detect deviations from normal operating conditions, indicating potential issues that need attention.
Degradation Analysis
Degradation analysis involves studying the wear and tear of assets over time. It helps in understanding how assets deteriorate and the factors influencing this process. Benefits include:
- Remaining Useful Life (RUL) Estimation: Estimating the remaining life of an asset helps in planning maintenance and replacements more effectively.
- Optimal Maintenance Scheduling: By knowing how quickly assets degrade, maintenance can be scheduled at optimal intervals, balancing cost and performance.
How to Leverage Analytics for Asset Condition Assessment
Implementing analytics for asset condition assessment involves several steps:
- Data Collection: Gather data from various sources such as sensors, inspection reports, and historical records. Ensure data quality and consistency.
- Data Integration: Integrate data from different sources into a centralised system. This creates a comprehensive view of asset conditions and performance.
- Model Development: Develop machine learning models tailored to your assets and operating environment. Use historical data to train these models for predictive maintenance and anomaly detection.
- Degradation Analysis: Conduct regular degradation studies to understand asset wear and tear. Use this information to refine maintenance schedules and improve asset management strategies.
- Implementation and Monitoring: Implement the models and tools in your asset management processes. Continuously monitor asset conditions and update models with new data to improve accuracy.
- Decision Making: Use insights gained from analytics to make informed decisions about maintenance, replacements, and asset utilisation. Prioritise actions based on risk and performance impact.
Conclusion
Understanding asset condition is indispensable for managing risk and enhancing performance. By leveraging advanced analytics such as machine learning and degradation analysis, organisations can transition from reactive to proactive asset management. This not only ensures operational efficiency and safety but also drives cost savings and extends the life of assets. Embracing these technologies and methodologies empowers organisations to make data-driven decisions, ultimately fostering a resilient and robust asset management strategy.